Question
Can predictive analytics enhance resource allocation and advance health equity for underserved communities?
At a Glance
This article discusses the pervasive health inequity and resource misallocation in underserved communities. We can effectively reallocate healthcare resources to improve health outcomes by harnessing predictive analytics. This transition is a collaborative effort involving stakeholders ranging from healthcare providers to policymakers. We can make healthcare more equitable for all by overcoming barriers to change.
Major Points to Consider
- Persistent Health Inequity: Despite medical advancements, underserved communities still suffer from unequal distribution of healthcare resources.
- Predictive Analytics as a Solution: This data-driven approach offers a proactive method to allocate resources strategically, identifying high-risk individuals and redirecting healthcare resources efficiently.
- Stakeholder Involvement: Achieving health equity through predictive analytics requires the collaborative efforts of healthcare providers, administrators, policymakers, and community members.
- Challenges: Implementing predictive analytics poses challenges in data integrity, interdisciplinary collaboration, ethical considerations, and adaptability.
- Transformative Opportunities: These challenges can be turned into opportunities through community-centric design, strategic data partnerships, integrative training programs, and more.
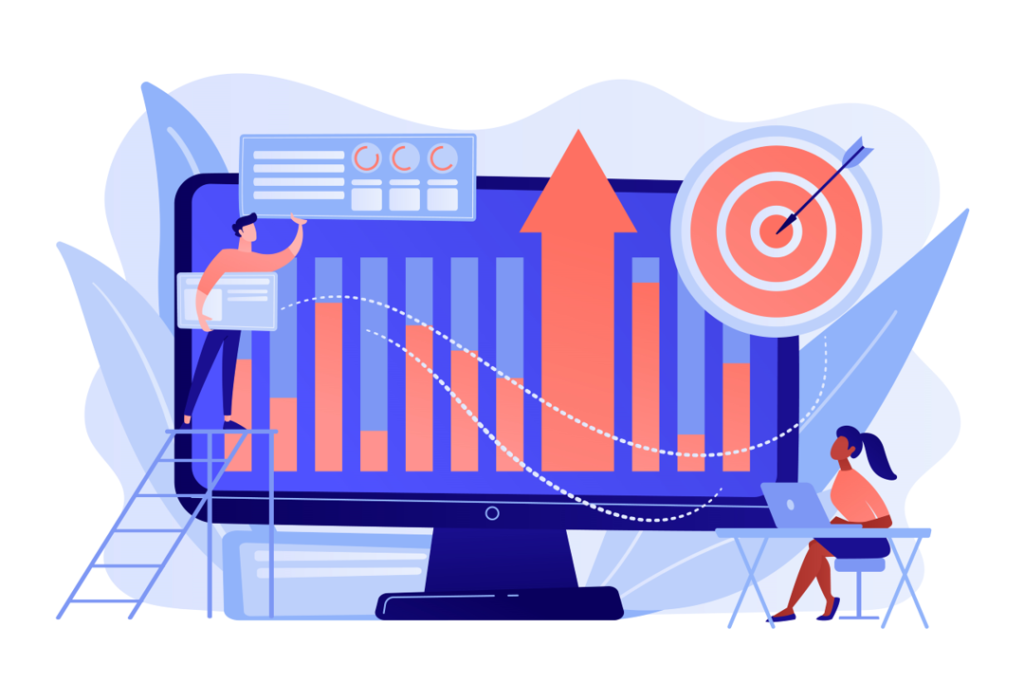
In an age of monumental medical science and technology advancements, persistent health inequities in underserved communities are a striking reminder of deep-rooted systemic challenges in our healthcare system. These inequities, perpetuated by the unequal distribution of resources, do more than highlight logistical oversights; they paint a grim picture of the healthcare divide in the United States.
Health Inequity and Resource Misallocation
As we strive to advance health equity, the inequitable resource allocation in underserved communities must take center stage, especially in ‘healthcare deserts.’ These areas, representing over 80% of U.S. counties, are critically deprived of essential health services, including primary care providers, pharmacies, hospitals, hospital beds, trauma centers, and low-cost health centers. Moreover, many residents in these communities face daunting personal and financial barriers that exacerbate health disparities.
The consequences of inequitable resource distribution extend beyond health outcomes. As spotlighted by the Community Health and Economic Prosperity (CHEP) initiative, spearheaded by the Office of the Surgeon General, the United States lags behind other high-income OECD countries in critical health metrics, including life expectancy, obesity, and infant mortality. This burdens the economy and impedes community growth and development. Communities deprived of sufficient healthcare resources have higher unemployment rates and lower economic productivity impacted by the disproportionate increases in chronic diseases and behavioral health disorders. These challenges often stem from insufficient preventive care investments and unequal economic opportunities, especially in underserved communities.
Leveraging Predictive Analytics
Recognizing the complex interplay between health inequities and resource imbalances in underserved communities, leveraging innovative solutions that target these challenges is imperative. Predictive analytics offers a proactive way to forecast community health needs and strategically allocate resources, ensuring these communities are not perpetually marginalized. Harnessed effectively, predictive analytics can drive better decision-making, allowing healthcare organizations serving these communities to pivot from merely reacting to immediate health challenges to proactively addressing them.
A pivotal study by Schulte and his team employed predictive analytics to identify individuals at high risk of hospitalizations due to conditions that could be managed outside a hospital setting, termed ‘ambulatory care-sensitive conditions.’ They analyzed real-world claims data and spotlighted the precision and effectiveness of predictive methodologies by comparing the machine learning-based Random Forest model to a traditional statistical logistic regression model. The machine learning model had greater accuracy, and this study demonstrated that early, data-driven interventions can help redirect and optimize healthcare resources.
Translating this to the context of underserved communities where resources are limited, the application of predictive models can be transformational. Healthcare organizations can strategically allocate resources to provide crucial, proactive care by leveraging such models to detect high-risk groups. This optimized resource utilization aligns with the healthcare system’s transition toward value-based care, emphasizing improved health outcomes, patient satisfaction, and cost-effectiveness.
Stakeholders in Predictive Health Equity
The path to achieving health equity through predictive analytics is multifaceted and requires the concerted efforts of diverse stakeholders. Their roles are pivotal in translating predictive analytics into tangible benefits for underserved communities, particularly when addressing the challenge of resource allocation.
Healthcare Providers: Often serving as the initial touchpoint for patients, healthcare providers play a pivotal role in shaping healthcare outcomes in underserved communities. With insights from predictive tools, providers can precisely forecast the demand for specific medical supplies, specialists, or targeted interventions. By synergizing their clinical expertise with data analytics, they can pinpoint and prioritize the essential resources to address prevailing health disparities.
Administrators: The onus falls on administrators to manage and distribute resources effectively within healthcare organizations. Predictive analytics offers them a precise tool to gauge future demands, allowing for a more efficient allocation of resources in underserved areas. By understanding patterns, administrators can better allocate resources where they’re needed most, ensuring that facilities in these communities are well-resourced.
Policymakers: Equipped with insights from predictive analytics, policymakers can reshape the landscape of healthcare resource allocation. Data-driven insights can inform them about the specific resource needs of underserved communities, allowing for the formulation of policies that directly address these gaps. It could lead to more targeted funding, the establishment of specialized clinics, or even the redirection of medical training programs to focus on prevalent health issues in these areas.
Communities: The communities themselves are at the core of any successful health intervention. Direct inputs from community members are fundamental in refining data-driven models. By interlacing their lived experiences with insights from predictive analytics, we attain a comprehensive view of resource needs. Beyond data, their engaged and proactive involvement ensures that every resource decision directly addresses the most pressing, community-specific challenges.
Challenges of Integrating Predictive Analytics
The potential of predictive analytics to revolutionize resource allocation in underserved communities is undeniable. However, just like any groundbreaking approach, it brings challenges that must be strategically addressed to ensure optimal deployment.
Data Integrity and Quality: A predictive model is only as good as the data that feeds it. One of the core challenges in underserved communities is the availability and reliability of comprehensive health data. Incomplete records, unstructured data sources, and inconsistencies can skew predictions, potentially leading to misallocated resources. For this transformative model to yield dividends, organizations must emphasize collecting high-quality, diverse data that reflects the targeted communities’ unique health profiles and challenges.
Interdisciplinary Collaboration: The real-world application of predictive analytics transcends traditional medical boundaries. It involves intertwining domains, from healthcare professionals to IT specialists and data scientists, all working in unison. This multi-disciplinary approach requires a concerted effort to break silos and foster an environment where various experts collaboratively pinpoint resource allocation solutions tailored to community needs.
Ethical Considerations: Harnessing vast amounts of health data creates ethical dilemmas, especially around privacy, consent, and potential misuse. Ensuring data privacy while maintaining the efficiency of the predictive model is a delicate balance that healthcare organizations must navigate. Moreover, the predictions should be viewed empathetically, ensuring individuals or groups aren’t unintentionally stigmatized.
Continuous Adaptability: The dynamic nature of healthcare needs, especially in underserved communities, necessitates an agile predictive analytics model. This means that, rather than static, these models must be frequently revisited, refined, and adapted to mirror the ever-evolving healthcare landscape, ensuring resources are always channeled where they can make the most impact.
Transforming Challenges into Opportunities
By viewing the hurdles of integrating predictive analytics as potential pivot points, healthcare organizations can design innovative strategies and transform them into opportunities. The transformative potential of these challenges lies in the creative strategies that healthcare providers, administrators, policymakers, and communities can co-develop to build a more equitable future. Here are some key areas of opportunity:
Community-Centric Design
Traditional healthcare models often focus on broad demographics rather than the unique needs of individual communities. By placing the community at the center of the predictive analytics model, healthcare organizations can ensure that predictions are grounded in lived realities. Regular feedback loops can refine models, making them culturally sensitive and attuned to real-world challenges. This participatory approach builds trust, promotes community empowerment, and fosters transparency, which is essential for sustainable transformation. Tailored interventions address pressing needs and forge a vital connection with the community.
Strategic Data Partnerships
Data integrity and quality are central to predictive analytics. Standardizing and securing data collection requires collaboration between healthcare organizations, tech companies, academic institutions, and communities. Strategic partnerships provide consistent data reflecting unique health challenges in underserved populations. Investment in these alliances, focusing on trust, shared platforms, and common standards respecting privacy and ethics, ensures precise and actionable predictions. This commitment to data integrity leads to optimized resource allocation.
Integrative Training Programs
The demand for professionals adept in both medical and data analytics is critical. Developing training modules fusing these disciplines can bridge this skills gap. Educational and healthcare institutions must prioritize cross-disciplinary learning and collaboration, fostering seamless integration of predictive analytics into healthcare strategies. This integration forms agile teams capable of real-time decision-making that aligns with community needs, enhancing the precision and responsiveness of healthcare interventions.
Feedback and Iteration
Healthcare is complex and ever-changing. Embracing iterative predictive analytics involves continuous feedback, analysis, and strategy refinement. Active engagement with data and the communities served, alongside regular review and adaptation mechanisms, ensures ongoing relevance and impact. This agile method aligns with contemporary principles of continuous improvement, nurturing a culture of innovation and excellence that drives meaningful change.
Localized Policy Advocacy
Predictive analytics in healthcare intertwines technology with policy, ethics, and community engagement. As this field matures, healthcare organizations must proactively collaborate with policymakers, advocating for integration and addressing ethical concerns. Forming groups with medical professionals, data scientists, and community representatives can lead to legislation promoting interdisciplinary collaboration and ethical guidelines. This proactive stance ensures transparency and equity, guaranteeing that resource allocation is efficient and ethically aligned with the unique needs of underserved communities.
Conclusion
The stark health inequities and unequal distribution of healthcare resources in underserved communities necessitate a transformative approach. Predictive analytics provides an innovative solution, enabling healthcare organizations to move from reaction to proactive strategies that align with the broader transition toward value-based care. We can enhance health equity and appropriate resource allocation by considering the unique challenges of integrating predictive analytics and strategically turning them into opportunities.
References
Bauer U. E. (2019). Community Health and Economic Prosperity: An Initiative of the Office of the Surgeon General. Public health reports (Washington, D.C. : 1974), 134(5), 472–476. https://doi.org/10.1177/0033354919867727
Nguyen, A., & Kim, S. (2021). Mapping healthcare deserts: 80% of the country lacks adequate access to healthcare. Published on September 9. Retrieved from https://www.goodrx.com/healthcare-access/research/healthcare-deserts-80-percent-of-country-lacks-adequate-healthcare-access
Schulte, T., Wurz, T., Groene, O., & Bohnet-Joschko, S. (2023). Big Data Analytics to Reduce Preventable Hospitalizations-Using Real-World Data to Predict Ambulatory Care-Sensitive Conditions. International journal of environmental research and public health, 20(6), 4693. https://doi.org/10.3390/ijerph20064693